Towards a new generation of artificial intelligence in China
Artificial intelligence has become a main driving force for a new round of industrial transformation around the world. Many countries including China are seizing the opportunity of the AI revolution to promote domestic economic and technological development. This Perspective briefly introduces the New Generation Artificial Intelligence Development Plan of China (2015–2030) from the point of view of the authors, a group of AI experts from academia and industry who have been involved in various stages of the plan. China’s AI development plan outlines a strategy for science and technology as well as education, tackling a number of challenges such as retaining talent, advancing fundamental research and exploring ethical issues. The New Generation Artificial Intelligence Development Plan is intended to be a blueprint for a complete AI ecosystem for the country.
This is a preview of subscription content, access via your institution
Access options
Access Nature and 54 other Nature Portfolio journals
Get Nature+, our best-value online-access subscription
cancel any time
Subscribe to this journal
Receive 12 digital issues and online access to articles
133,45 € per year
only 11,12 € per issue
Buy this article
- Purchase on SpringerLink
- Instant access to full article PDF
Prices may be subject to local taxes which are calculated during checkout
Similar content being viewed by others
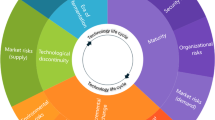
Building an AI ecosystem in a small nation: lessons from Singapore’s journey to the forefront of AI
Article Open access 02 July 2024
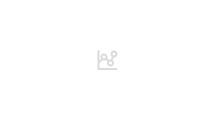
Why general artificial intelligence will not be realized
Article Open access 17 June 2020
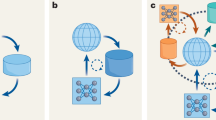
A social path to human-like artificial intelligence
Article 17 November 2023
References
- China issues guideline on artificial intelligence development. Gov.cnhttp://english.gov.cn/policies/latest_releases/2017/07/20/content_281475742458322.htm (2017).
- Pan, Y. Heading toward artificial intelligence 2.0. Engineering2, 409–413 (2016). ArticleGoogle Scholar
- Pan, Y.-h. Special issue on artificial intelligence 2.0. Front. Inform. Technol. Electron. Eng.18, 1–2 (2017). ArticleGoogle Scholar
- Pan, Y.-h. Special issue on artificial intelligence 2.0: theories and applications. Front. Inform. Technol. Electron. Eng.19, 1–2 (2018). ArticleGoogle Scholar
- Zhuang, Y.-t., Wu, F., Chen, C. & Pan, Y.-h. Challenges and opportunities: from big data to knowledge in AI 2.0. Front. Inform. Technol. Electron. Eng.18, 3–14 (2017). ArticleGoogle Scholar
- Peng, Y.-x et al. Cross-media analysis and reasoning: advances and directions. Front. Inform. Technol. Electron. Eng.18, 44–57 (2017). ArticleGoogle Scholar
- Li, W. et al. Crowd intelligence in AI 2.0 era. Front. Inform. Technol. Electron. Eng.18, 15–43 (2017). ArticleGoogle Scholar
- Zheng, N.-n et al. Hybrid-augmented intelligence: collaboration and cognition. Front. Inform. Technol. Electron. Eng.18, 153–79 (2017). ArticleGoogle Scholar
- Zhang, T. et al. Current trends in the development of intelligent unmanned autonomous systems. Front. Inform. Technol. Electron. Eng.18, 68–85 (2017). ArticleGoogle Scholar
- Fisk, P. Meituan Dianping: China’s everything-app to “eat better, live better”. Gamechangershttps://www.thegeniusworks.com/gamechanger/meituan-dianping/ (2019).
- China’s consumer credit balance expected to exceed 10t yuan by 2020. China Banking Newshttp://www.chinabankingnews.com/2019/01/21/chinas-consumer-credit-balance-expected-to-exceed-10t-yuan-by-2020/ (2019).
- The Mobile Economy 2020 (GSMA Intelligence, 2020).
- Global Status Report on Road Safety 2018 (World Health Organization, 2018).
- Chen, W. et al. Cancer statistics in China, 2015. CA: Cancer J. Clin.66, 115–132 (2016).
- Bi, W. L. et al. Artificial intelligence in cancer imaging: clinical challenges and applications. CA: Cancer J. Clin.69, 127–157 (2019).
- LeCun, Y., Bengio, Y. & Hinton, G. Deep learning. Nature521, 436–444 (2015). ArticleGoogle Scholar
- Hosny, A., Parmar, C., Quackenbush, J., Schwartz, L. H. & Aerts, H. J. W. L. Artificial intelligence in radiology. Nat. Rev. Cancer18, 500–510 (2018). ArticleGoogle Scholar
- Bejnordi, B. E. et al. Diagnostic assessment of deep learning algorithms for detection of lymph node metastases in women with breast cancer. JAMA318, 2199–2210 (2017). ArticleGoogle Scholar
- Papandreou, G. et al. Weakly-and semi-supervised learning of a deep convolutional network for semantic image segmentation. In Proc. IEEE Int. Conf. Computer Vision 1742–1750 (IEEE, 2015).
- Bonawitz, K. et al. Towards federated learning at scale: system design. Preprint at https://arxiv.org/abs/1902.01046 (2019).
- Song, J. et al. IEEE Trans. Neural Netw. Learn. Syst.https://doi.org/10.1109/tnnls.2020.2989364 (2020).
- Growing IoT in China (GSMA, 2019).
- Zhuang, Y. et al. The next breakthroughs of artificial intelligence: the interdisciplinary nature of AI. Engineering6, 245–247 (2020). ArticleGoogle Scholar
- Wu, W., Huang, T. & Gong, K. Ethical principles and governance technology development of AI in China. Engineering6, 302–309 (2020). ArticleGoogle Scholar
- Amini, A. Soleimany, A. P., Schwarting, W., Bhatia, S. N. & Rus, D. Uncovering and mitigating algorithmic bias through learned latent structure. In Proc. 2019 AAAI/ACM Conf. AI, Ethics, and Society 289–295 (ACM, 2019).
- Papernot, N., Abadi, M., Erlingsson, U., Goodfellow, I. J. & Talwar, K. Semi-supervised knowledge transfer for deep learning from private training data. In 5th Int. Conf. Learning Representations (ICLR, 2017).
- China AI Development Report 2018 (Tsinghua Univ., 2018).
- Lv, Y.-G. Artificial intelligence: enabling technology to empower our society. Engineering6, 205–206 2020). ArticleGoogle Scholar
- Roberts, H. et al. The Chinese approach to artificial intelligence: an analysis of policy and regulation. Preprint at https://doi.org/10.2139/ssrn.3469784 (2019).
Acknowledgements
We thank H. Shum and Z. Zhang for discussions and comments. We also thank the strategic consulting research project of the Chinese Academy of Engineering ‘AI 2.0 in China’, and the disruptive information technology research group of the Department of Information and Electronic Engineering at the Chinese Academy of Engineering. This paper is partly supported by AI Young Scientists Alliance, STCSM(Xuhui), SHEITC, NSFC (61625107).
Author information
- These authors contributed equally: Fei Wu, Cewu Lu, Mingjie Zhu.
Authors and Affiliations
- Zhejiang University,, Hangzhou, China Fei Wu, Xi Li, Yueting Zhuang & Yunhe Pan
- Shanghai Jiao Tong University, Shanghai, China Cewu Lu
- CraiditX, Shanghai, China Mingjie Zhu & Kai Yu
- Imsight Medical Technology, Hong Kong, China Hao Chen
- Tsinghua University, Beijing, China Jun Zhu
- ByteDance, Beijing, China Lei Li
- Nanjing University, Nanjing, China Ming Li
- Hong Kong University of Science and Technology, Hong Kong, China Qianfeng Chen
- Momenta, Beijing, China Xudong Cao
- Meituan, Beijing, China Zhongyuan Wang
- University of Science and Technology of China, Hefei, China Zhengjun Zha
- Zhejiang Laboratory, Hangzhou, China Yunhe Pan
- Fei Wu